A friend and I were talking recently about the dramatic change that digital transformation will create. The potential impact on our lives is tremendous, from autonomous vehicles that whisk us to and from work, to drones that deliver goods directly to our homes or...
Overlooked in the digital transformation: Ethical, Societal, Legal, Regulatory issues
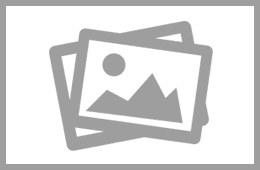