In my last post I tried to illustrate the importance (and the challenges) of data to digital transformation. This is often a complex and difficult idea for people to understand - why is "data" so hard? Why can't computer systems work more effectively? ...
Digital Transformation, Data and Innovation
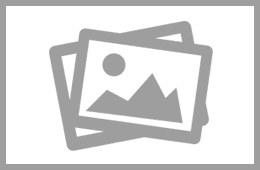